The dark side of urban Artificial Intelligence: addressing the environmental and social impact of algorithms
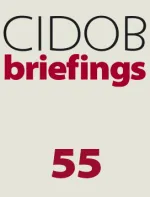
This CIDOB briefing summarises the key findings of the international seminar “The dark side of urban artificial intelligence: addressing the environmental and social impact of algorithms”, held on June 19th, 2023 at CIDOB and organised by CIDOB’s Global Cities Programme with support from Barcelona City Council. Scholars, experts and practitioners convened to deliberate and offer recommendations for the efficient governance and deployment of algorithmic tools in urban settings, with a view to mitigating the environmental, social and political challenges associated with AI.
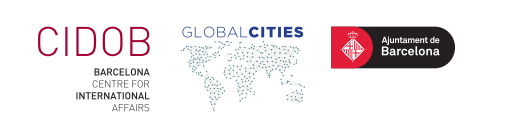
We are seeing a surge in global efforts to establish governance frameworks for Artificial Intelligence (AI): from the United States’ Executive Order on the Safe, Secure, and Trustworthy Development and Use of AI (October 2023), to the AI Safety Summit in the United Kingdom (November 2023); taking in a consolidated working draft Convention by the Council of Europe on AI, Human Rights, Democracy and the Rule of Law (July 2023); not to mention the political agreement reached in December 2023 (to be ratified in 2024) between the negotiators at the European Parliament and the Council to adopt the EU AI Act. According to the Council of Europe’s compendium of AI Initiatives, there are currently more than 600 ongoing initiatives aimed at governing AI.
However, what is to be governed when it comes to algorithms? According to a recent piece in The Economist, any AI regulation should first aim to answer three key questions: What should the world worry about? What should any rules target? And how should they be enforced? It is far from clear whether any of the above initiatives will hit the nail on the head in terms of AI’s impact on the environment and society. The issue becomes even thornier if we take into account the multi-stakeholder dimension of AI governance: it is not just national or local governments, or intergovernmental organisations who are involved. Private players – and especially companies – play a growing role, if not the leading one, in some of these initiatives.
This CIDOB briefing expands upon the findings of the international seminar “The dark side of urban artificial intelligence: addressing the environmental and social impact of algorithms”, held on June 19th, 2023. We delve into two crucial aspects of AI and algorithmic governance: firstly, the environmental consequences of AI, and secondly, the wider social and political implications of algorithms. The briefing wraps up by offering insights and recommendations for the effective governance of AI in urban contexts.
1. The environmental impact of algorithms: “AI for sustainability” and “sustainable AI”
There are two interconnected perspectives on the relationship between AI and environmental sustainability, broadly referred to as “AI for sustainability” and “sustainable AI” (van Wynsberghe, 2021).1 The former involves using algorithmic tools in areas that contribute to ecologically desirable developments, such as climate protection. Examples of AI applications in this field include counting trees, providing precise estimates of biodiversity in various areas, monitoring real-time weather patterns, or forecasting energy consumption, air quality and CO₂ emissions, as well as enhancing efficiency in resource allocation. These illustrate how AI serves as a robust tool for swift and informed decision-making, facilitating progress towards more sustainable cities. Given this, “AI for sustainability” accounts for the enthusiastic adoption of AI solutions by many cities. The AI4Cities project is a good illustration of this trend, representing one of the most significant initiatives showcasing how cities are actively seeking AI-driven solutions in the energy and mobility domains to support their transitions to carbon neutrality.
Yet, as more resources are dedicated to the development and use of urban AI solutions, it becomes increasingly crucial to consider the environmental impact of these technologies. Indeed, designing, producing and employing AI technologies requires a physical infrastructure that calls for extensive amounts of material resources, including water, metals, energy and human labour. Consequently, not only their computational power but their very material existence gives rise to significant ethical issues from a sustainability standpoint. After all, and as Falk and van Wynsberghe (2023, p.7) put it: “How useful can the impact of an AI system be towards sustainable ends if its development and use defeat the purpose of its existence in the first place?”
In this context, the term “AI for sustainability” should be distinguished from “sustainable AI”. The latter is about “developing, implementing, and using AI in a manner that minimises the adverse social, ecological and economic impacts of the applied algorithms” (Rohde et al., 2021, p.1). However, the environmental impact is not easy to analyse, let alone estimate. One key aspect involves grounding the discussion in the relationship between the benefits of AI systems and their environmental cost in factual data. The problem is that at present developers and operators of these systems are not furnishing the necessary data, hindering the formulation and implementation of effective policies. The most recent iterations of the EU’s AI Act represent a potential breakthrough. For the first time they may compel companies to measure and disclose information regarding the environmental impact of specific high-risk systems. This might entail incorporating data collection methods into these systems, drawing inspiration from already-established approaches for monitoring energy consumption, CO₂-equivalent emissions, water usage, mineral use for hardware, and electronic waste generation. This would streamline the assessment of the sustainability of AI systems (Mollen and Vieth-Ditlmann, 2023).
Yet, considering that the evaluation of the sustainability of AI is still a developing and nascent area, the SustainAI index can be regarded as another notable stride in this direction. It provides a comprehensive blueprint for assessing and improving the sustainability of AI systems. This initiative proposes evaluating the environmental sustainability of algorithms through different stages (planning and design, data, development and implementation) based on four criteria:2 energy consumption, greenhouse gas emissions, sustainability in use and indirect resource consumption. Among these, energy consumption (intertwined with greenhouse gas emissions) is acknowledged as the primary source of concern. Granted, all Internet-related activities rely heavily on substantial electricity, primarily sourced from fossil fuels. However, when compared with other technologies, AI, especially applications like ChatGPT, stands out for its extraordinary power usage.
To start with, training a large language or other AI model requires huge amounts of power. Furthermore, large language models rank among the biggest in the realm of machine learning, incorporating as many as hundreds of billions of parameters. The training process demands several weeks of GPU hours, contributing to carbon emissions. As an illustration, the energy consumption for training BLOOM, an open-access multilingual language model, equated to the amount needed to power an average American home for 41 years (Falk and van Wynsberghe, 2023, p.5). Moreover, the chatbot or any other end product needs electricity every time it is used. Recently, some proposals have emerged to address this concern, including the idea of affixing a label to algorithms that discloses the amount of CO₂ emissions and computing power used in their creation. For a city administration, prioritising these types of algorithms may be a good way of improving the ecological sustainability of their digital initiatives, as most urban technologies are not developed in-house. Likewise, local governments could give precedence to employing algorithms trained with small and conscientiously curated datasets. Although this approach may take more time, it not only contributes to sustainability but also enhances fairness and accuracy, thereby contributing to the reduction of “data pollution”.
Secondly, it is crucial to take into account the broader infrastructure that supports and links hardware, encompassing the energy consumption of networking systems, maintenance of data centres and cooling systems (Falk and van Wynsberghe, 2023, p.5). This includes the production of computer chips and the establishment of data centres where AI operates. Fortunately, there are existing initiatives aimed at rendering urban data centres more eco-friendly, such as Stockholm Data Parks (see box 1) or a Paris project using server energy to heat swimming pool water. However, there is a pressing need for broader efforts at the urban level, as these measures remain more anecdotal than standard practice.
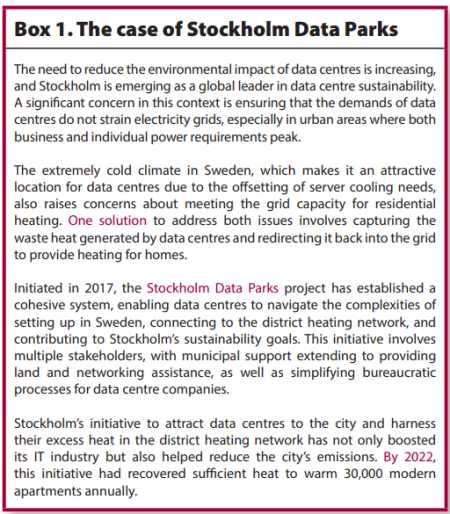
2. The social impact of algorithms
As is the case for the environmental impact of AI, two main views prevail when it comes to the political dimensions of algorithms, which could be labelled “AI for democracy” and “democratic AI” (see box 2). Likewise, and while the political and social impact of AI may have been researched for longer (e.g., disinformation and misinformation or algorithmic discrimination), its actual implications for politics and societies are still far from clear. In this regard, most concerns seem to focus on the possibility of the singularity, i.e., the point in time at which AI surpasses human intelligence. However, and as Shazade Jameson put it during the seminar, “the real revolution in AI will be mundane”. Indeed, probably the most problematic aspect of (generative) AI is that it hides in plain sight.
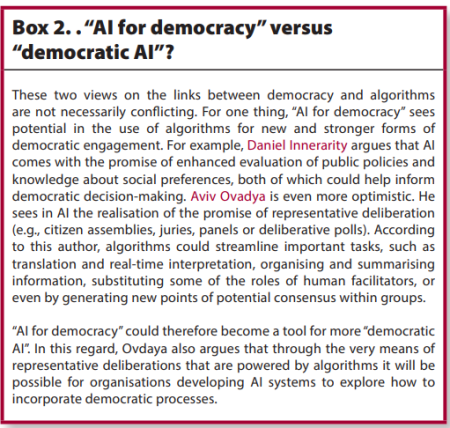
Algorithms are already embedded in many of our daily habits, from searching for directions on maps or mobile navigation apps to voice assistants. Not to mention that most online services rely on AI: generally, what we see online is the result of classification and association algorithms (such as search engines or online advertising), and we may be filtered by an algorithm without us even knowing (when applying for a job, a mortgage, or enrolling for medical and insurance programmes).
Public administrations also use algorithms extensively, for example, for medical diagnosing, policing, to find eligible persons for public subsidies, or to decide on whether to provide police protection to survivors of gender violence. At the local level, many municipalities are using generative AI models to gain insights from unstructured data, improving the understanding of what is happening in the city, as well as using algorithmic tools to enable public services delivery to be more accessible and efficient (typically taking the form of chatbots).
There are several shortcomings in each of these cases, and they all usually boil down to issues of discrimination, transparency, accuracy and trustworthiness. Examples abound. In 2013, it was found that Google searches using “black-sounding” names were more likely to turn up ads for services such as criminal background checks; in 2015, Amazon realised its new system was not rating candidates for software developer jobs and other technical posts in a gender-neutral way; in 2018, it was found that setting a user’s gender to female in Google resulted in being shown fewer ads for high-paying jobs.
However, private corporations are not alone when it comes to the use of algorithms that discriminate based on gender and/or race. There are also several cases where policing algorithms have been found to discriminate against people based on where they lived, such as in Chicago in the United States and Durham in the UK. More recently, Eticas Foundation and Fundación Ana Bella-Red de Mujeres Supervivientes found that the algorithm used in the Spanish Ministry of the Interior’s system to assess the risks of survivors from gender violence, VioGén, falls short of delivering on its promise. Some 80% of interviewed survivors raised issues with the use of the algorithm. In the Dutch city of Rotterdam, an algorithm used to rank welfare recipients based on their fraud risk was found to discriminate against single mothers.
These shortcomings are magnified because algorithms are usually in the hands of a small number of private companies, in what has been referred to by Aviv Ovadya as “autocratic concentration”. These private, for-profit actors cannot by themselves address the costs and drawbacks of AI. In many cases, not even AI researchers fully understand the recommendations made by algorithms.
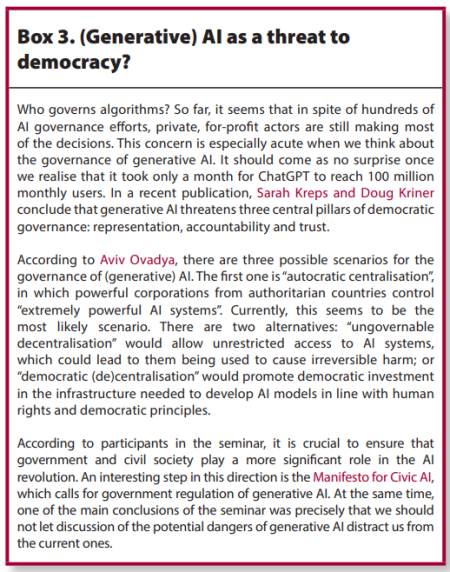
3. Lessons learned and challenges ahead for local governments
a) Regulation and governance come with their own challenges
It is widely acknowledged that addressing the challenges posed by AI often involves seeking solutions through regulatory measures. However, regulating AI comes with its own set of difficulties, including dealing with the rapid pace of AI advancements, dissecting the elements to be regulated and deciding who regulates and how. At the global level, the current geopolitical conditions add an extra layer of complexity to the task of regulation.
From a European standpoint, the continent is facing the consequences of excessive regulation, which has led to gaps in investment in research and development as well as capacity-building. Similarly, the seminar’s participants expressed concerns that AI requirements imposed by Europe can pose significant challenges to small businesses and open-source projects. Hence, while acknowledging the positive aspects of the EU’s AI Act, such as its human rights-based approach, it is essential to recognise the potential long-term negative impacts of the act and address them.
While many countries have already released national guidelines on AI, most local governments are still lagging behind on the development of regulatory frameworks for AI in a technical and policy capacity. While governance may certainly be a challenging task, four essential lessons to guide progress emerged from the seminar:
- Focus on processes: while most governance initiatives address AI outcomes, it is crucial to recognise that (machine) learning is a continuous process, and it is as part of these processes that policies should step in.
- Governing uncertainty is a constant practice: effectively governing uncertainty requires ongoing efforts, with feedback mechanisms and a supportive culture playing vital roles in enabling organisations, including local governments, to adapt over time.
- Accountability for algorithmic decisions should be established.
- Aim big, start small: optimal governance is built through projects, exemplified by initiatives like the Data Governance Clinics project. This innovative approach aligns data governance with the public interest in cities and underscores the importance of adopting an ambitious yet gradual approach to achieve broader goals.
b) Human resources: capacity-building and talent attraction
Decision-makers need to assess the available human resources capable of designing, implementing, deploying and overseeing urban AI systems. To fully reap the benefits of digital transformation, public sector leaders must acquire new skills that equip them to tackle the intricate challenges of the digital era. Artificial Intelligence is no exception to that, and the effective adoption and regulation of algorithmic tools require digital literacy among civil servants. These competencies encompass the ability to create enabling frameworks, foresee technological trends, implement measures to address ethical and human rights risks, comprehend the development of digital platforms, and collaborate effectively with third parties, including vendors. In essence, talent and digital skills are indispensable, underscoring the importance of enhancing the government’s digital capacity as a prerequisite for ambitious local AI projects. In the context of an urban AI strategy, capacity-building refers to the process of cultivating and reinforcing the skills, instincts, abilities, processes and resources that a local community requires to plan, design and deploy AI applications.
Interestingly, most local governments perceive capability constraints as a significant obstacle to both the adoption and regulation of AI applications. More specifically, cities commonly encounter two types of limitations: the availability of a local workforce with the requisite skills for constructing and managing the AI system (human capacity), and the proficiency of this workforce in interacting with and supervising the AI system (AI literacy). These limitations are linked to a scarcity of locally accessible skills and a global shortage of AI talent. It is worth noting that in the global race for IT talent and specific AI skills, the private sector has traditionally surpassed governments in their ability to attract specialised human resources. Consequently, many cities lack the financial resources to develop urban technologies in-house, leading them to rely on outsourcing and procurement to access the technical expertise essential for AI development and governance.
The skills gap is not inconsequential. In the first place, poor knowledge among decision-makers responsible for funding AI solutions and those tasked with implementing the technology renders system monitoring very difficult. From a geographical perspective, the global competition for talent aggravates the imbalance between small and large cities. It should be noted that in secondary cities, the lack of capacity is often not solely technical but also legal, as they may lack the competences to develop technology. This heightens the risk of creating disparities between first and second-class cities. While the global digital divide and a city’s socioeconomic situation may exacerbate the shortage of AI skills in public administrations, this issue is a concern for affluent and economically challenged cities alike.
Cities can implement a series of measures to address these constraints. Foremost among these is the need to make capacity-building a central component of any effective local AI strategy. This involves investing in developing both technical capabilities (such as digital literacy) and interdisciplinary skills (such as AI regulation and law, AI ethics and AI business development). Ultimately, local governments must ensure that staff directly involved in implementing an AI system in an urban sector are adequately trained and informed about the specific AI system they are employing. This means they should have a comprehensive understanding of how AI may impact their responsibilities and be capable of interpreting the system’s output to identify potential failures. Moreover, any capacity-building strategy should also include specific efforts to educate the public about AI, its transformative effects on current practices, and the opportunities, challenges and risks it presents. Nevertheless, capacity-building initiatives alone may prove insufficient, prompting local governments to formulate strategies for attracting and retaining talent. In the immediate term, they can tackle budget and skills shortages by forging cross-sectoral collaborations with local stakeholders to offset the scarcity of public capacities.
c) Procurement is key
As argued above, most cities lack the internal capacity to develop AI solutions on their own, leading them to acquire urban AI primarily through procurement channels. In fact, AI procurement serves as a powerful governance tool that can be leveraged to address some of the harmful effects that AI use may have on citizens, especially those in vulnerable communities. However, throughout the procurement process, cities must possess the ability to assess the AI solutions presented to them. One effective method to ensure that private providers adhere to the city’s standards regarding digital rights and ethical principles is by incorporating procurement clauses.
For instance, in 2021 the City of Amsterdam formulated a set of contractual terms outlining the specific information required from suppliers. Municipal governments can keep control over the technology they adopt by seeking three types of information: technical transparency (the code), procedural transparency (the algorithm’s purpose and how it reaches its outcomes), and “explainability” (the rules that apply if an algorithm impacts someone personally). The value of these contractual terms lies in their ability to help local governments operationalise standards, create obligations and define responsibilities for trustworthy, transparent and accountable development and procurement of AI technologies. Unsurprisingly, other local governments, including Barcelona, are emulating Amsterdam’s approach by crafting their own AI procurement clauses.
d) Citizen participation and co-creation to enhance diversity
A fourth element that is crucial to address the adverse effects of AI is to engage civil society in both the development and use of algorithmic tools. The concerns surrounding AI extend beyond the principle of “public money, public ownership” to encompass public intelligence and citizen data. In that sense, seminar participants concurred that involving civil society in AI initiatives is essential to prevent biases from being ingrained in AI and to ensure that AI regulations are understandable to the general public.
Additionally, it was emphasised that when public entities use algorithms, consideration should be given to governance and institutional frameworks, recognising that the current state of data governance in public governance is far from optimal. Similarly, some participants underscored the importance of transparent research and public repositories, advocating for the implementation of mechanisms that hold public administrations accountable to their citizens when using automated decision systems.
References
Rohde, Friederike; Gossen, Maike; Wagner, Josephin and Santarius, Tilman (2021) “Sustainability challenges of Artificial Intelligence and Policy Implications”. Ökologisches Writschaften, 36.
van Wynsberghe, Aimee (2021) “Sustainable AI: AI for sustainability and the sustainability of AI”. AI Ethics, 1, 213-218.
Falk, Sophia and van Wynsberghe, Aimee (2023) “Challenging AI for Sustainability: what ought it mean?”. AI Ethics
Mollen, Anne and Vieth-Ditlmann, Kilian (2023) “Just Measure It: The Environmental Impact of AI”. SustainAI Magazine, number 3. Autumn, 2023.
Notes:
- The notion of sustainability is a complex one. It is often understood as comprising three distinct dimensions: an environmental, a social, and an economic one. In this section, we limit the analysis to the environmental dimension of sustainability.
- For a more detailed account of the different criteria, see: https://sustain.algorithmwatch.org/en/step-by-step-towards-sustainable-ai/
- For more examples on the use of algorithms in cities, refer to the Atlas of Urban AI curated by the Global Observatory of Urban Artificial Intelligence (led by CIDOB’s Global Cities Programme).